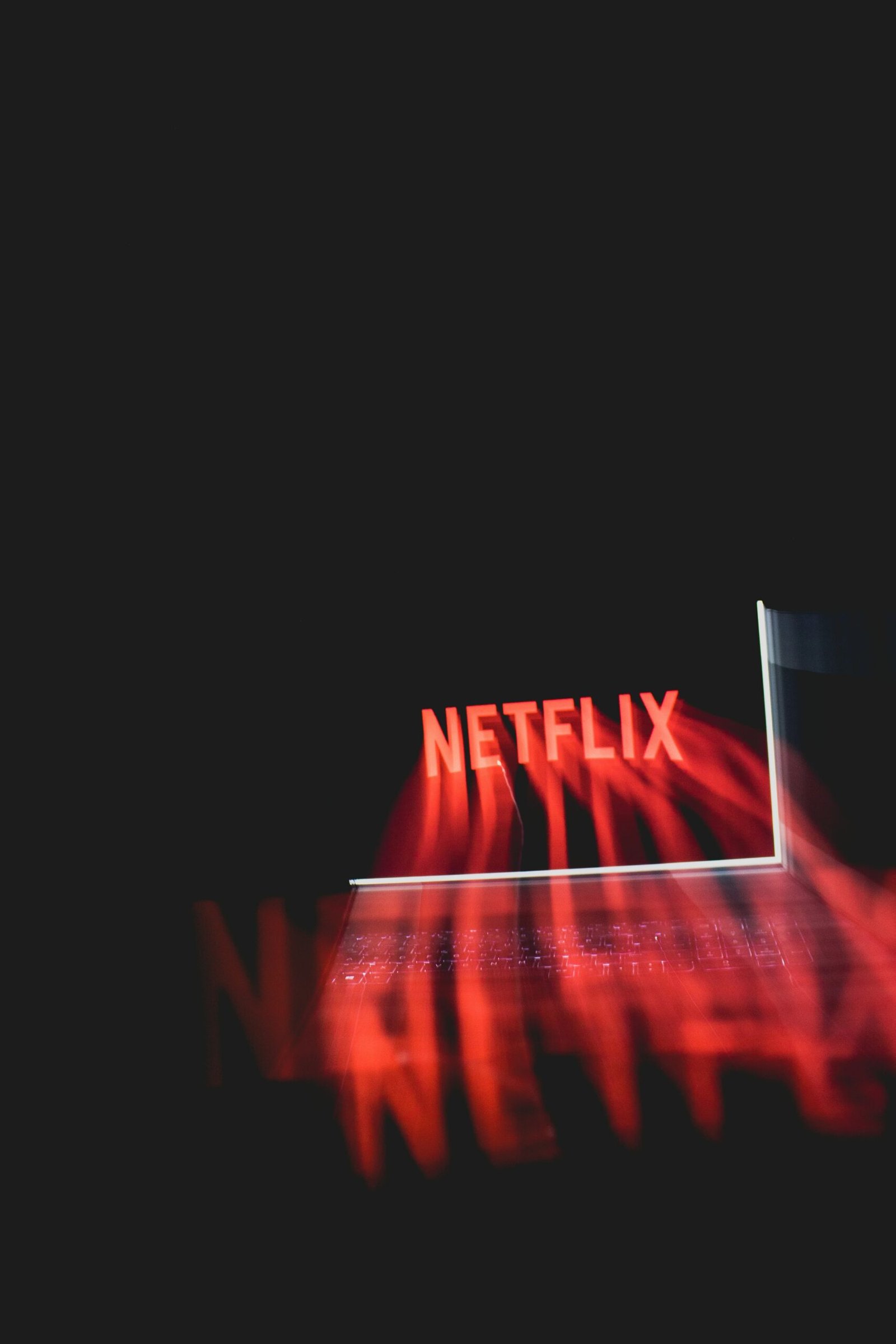
Introduction to Netflix’s Recommendation Engine
Netflix’s recommendation engine is a sophisticated algorithm designed to enhance user experience by providing personalized content suggestions. As a crucial component of the platform, this recommendation system plays a significant role in retaining subscribers by ensuring they find content that matches their preferences and viewing habits. The necessity of such a system becomes clear when considering the scale at which Netflix operates, serving millions of users globally.
The primary goal of Netflix’s recommendation engine is to sift through an extensive library of movies, TV shows, and documentaries to present each user with a tailored list of content. Personalized recommendations are vital in an era where digital content is abundant, and user attention spans are limited. By offering users content that aligns with their tastes, Netflix not only enhances the viewing experience but also encourages longer engagement periods and reduces the likelihood of subscription cancellations.
At the heart of Netflix’s recommendation engine lies a complex blend of algorithms and data analytics. These algorithms analyze a wide range of data points, including a user’s viewing history, ratings, and even the time of day they are most active on the platform. This data-driven approach allows Netflix to predict what content will be of interest to each user, thereby increasing the chances of user satisfaction and continued subscription.
The effectiveness of Netflix’s recommendation engine is evidenced by the platform’s high user retention rates. A well-functioning recommendation system is not just a luxury but a necessity for a streaming service operating on such a massive scale. By continually refining and optimizing its recommendation algorithms, Netflix ensures that it remains a leader in the streaming industry, providing a seamless and enjoyable viewing experience for its millions of users worldwide.
Algorithms and Data Science Behind the Recommendations
Netflix’s recommendation engine is a sophisticated amalgamation of several advanced algorithms and data science techniques, all aimed at providing a personalized and engaging user experience. At the core of this system are collaborative filtering, content-based filtering, and deep learning models. Each of these methods plays a crucial role in understanding and predicting user preferences.
Collaborative filtering is a technique that leverages the collective behavior of users to make recommendations. By analyzing the viewing patterns and ratings of users with similar tastes, Netflix can suggest content that aligns with an individual’s preferences. This method is particularly effective in identifying latent patterns and trends within large datasets.
Content-based filtering, on the other hand, focuses on the attributes of the content itself. By examining metadata such as genre, director, cast, and even more intricate features like dialogue and visual style, Netflix can recommend titles that share similar characteristics with content the user has previously enjoyed. This approach ensures that recommendations remain relevant even when user behavior changes.
Deep learning models further enhance the recommendation engine’s accuracy and efficiency. These models are designed to process vast amounts of data, including viewing history, ratings, and user interactions, to uncover complex relationships and patterns. Netflix employs various neural networks and machine learning techniques to continually refine these models, ensuring that recommendations improve over time.
The effectiveness of Netflix’s recommendation engine is also a testament to the expertise of its machine learning engineers and data scientists. These professionals are responsible for developing, training, and fine-tuning the algorithms that power the system. They work with enormous datasets, employing state-of-the-art tools and methodologies to ensure that the recommendations are both accurate and personalized.
In summary, the combination of collaborative filtering, content-based filtering, and deep learning models, supported by the expertise of skilled data scientists and machine learning engineers, enables Netflix to deliver highly effective and personalized recommendations to its users.
Personalization and User Experience
Netflix’s recommendation engine is a cornerstone of its user experience, providing personalized content suggestions that significantly enhance viewer satisfaction and engagement. Central to this personalization are various types of recommendations tailored to each user. Some of the most familiar categories include ‘Top Picks for You,’ which curates a list of titles based on the user’s viewing history and preferences, and ‘Because You Watched,’ which suggests content similar to what the user has previously enjoyed. Additionally, genre-specific recommendations highlight movies and shows within particular categories that align with the user’s tastes.
The effectiveness of these personalized recommendations stems from Netflix’s sophisticated algorithms, which analyze a myriad of data points. This includes individual viewing habits, such as the type of content watched, the genres preferred, and the duration of viewing sessions. Even the time of day when a user is most active can influence recommendations. For instance, Netflix might suggest lighter, more easily digestible content during the daytime and more complex series or movies in the evening when users are likely to have more time to engage deeply.
Netflix’s ability to tailor content so precisely relies on a combination of collaborative filtering, content-based filtering, and deep learning techniques. Collaborative filtering looks at patterns within user data to identify similarities between users and their preferences, while content-based filtering focuses on the attributes of the content itself. Deep learning models further enhance these methods by handling vast amounts of data and uncovering intricate patterns that simpler algorithms might miss.
This high degree of personalization has a profound impact on user satisfaction and engagement. Users are more likely to find content that aligns with their interests, reducing the time spent searching and increasing the time spent watching. This continuous cycle of personalized recommendations not only keeps users engaged but also fosters a sense of connection and loyalty to the platform. Ultimately, Netflix’s recommendation engine is not just a feature but a critical component of its strategy to enhance user experience and retain its subscriber base.
Challenges and Future Directions
Netflix’s recommendation engine, while highly effective, faces several challenges that need continual addressing. One significant issue is data privacy. As Netflix collects a vast amount of data on user preferences and viewing habits, ensuring the protection of this data is paramount. Stricter data privacy regulations globally, such as the General Data Protection Regulation (GDPR) in the European Union, necessitate robust data security protocols and transparent data usage policies. Netflix must navigate these regulations carefully to maintain user trust and comply with legal requirements.
Another challenge is algorithm bias. Recommendation systems can sometimes reinforce existing preferences, leading to a lack of diversity in content suggestions. This can result in users missing out on potentially interesting content outside their usual viewing patterns. To address this, Netflix continuously refines its algorithms to balance personalized recommendations with a diverse array of content, thereby enhancing the user experience.
The cold start problem is another hurdle for Netflix, particularly for new users. When a new user joins the platform, the recommendation engine initially lacks sufficient data to provide personalized suggestions. To mitigate this, Netflix uses hybrid recommendation approaches, combining collaborative filtering with content-based methods. By leveraging initial user interactions and general viewing trends, the platform can quickly generate more accurate recommendations.
Looking ahead, the future of Netflix’s recommendation engine will likely involve advanced artificial intelligence (AI) and machine learning technologies. Enhanced AI capabilities could enable more nuanced user profiling, capturing subtler preferences and viewing behaviors. This would allow for even more precise and personalized recommendations. Additionally, integrating natural language processing (NLP) could improve content discovery by understanding user queries and reviews more effectively.
As the streaming market becomes increasingly competitive, Netflix aims to stay at the forefront through continuous innovation in its recommendation strategies. By addressing current challenges and embracing future technological advancements, Netflix can maintain its position as a leading platform, offering users an unparalleled viewing experience.