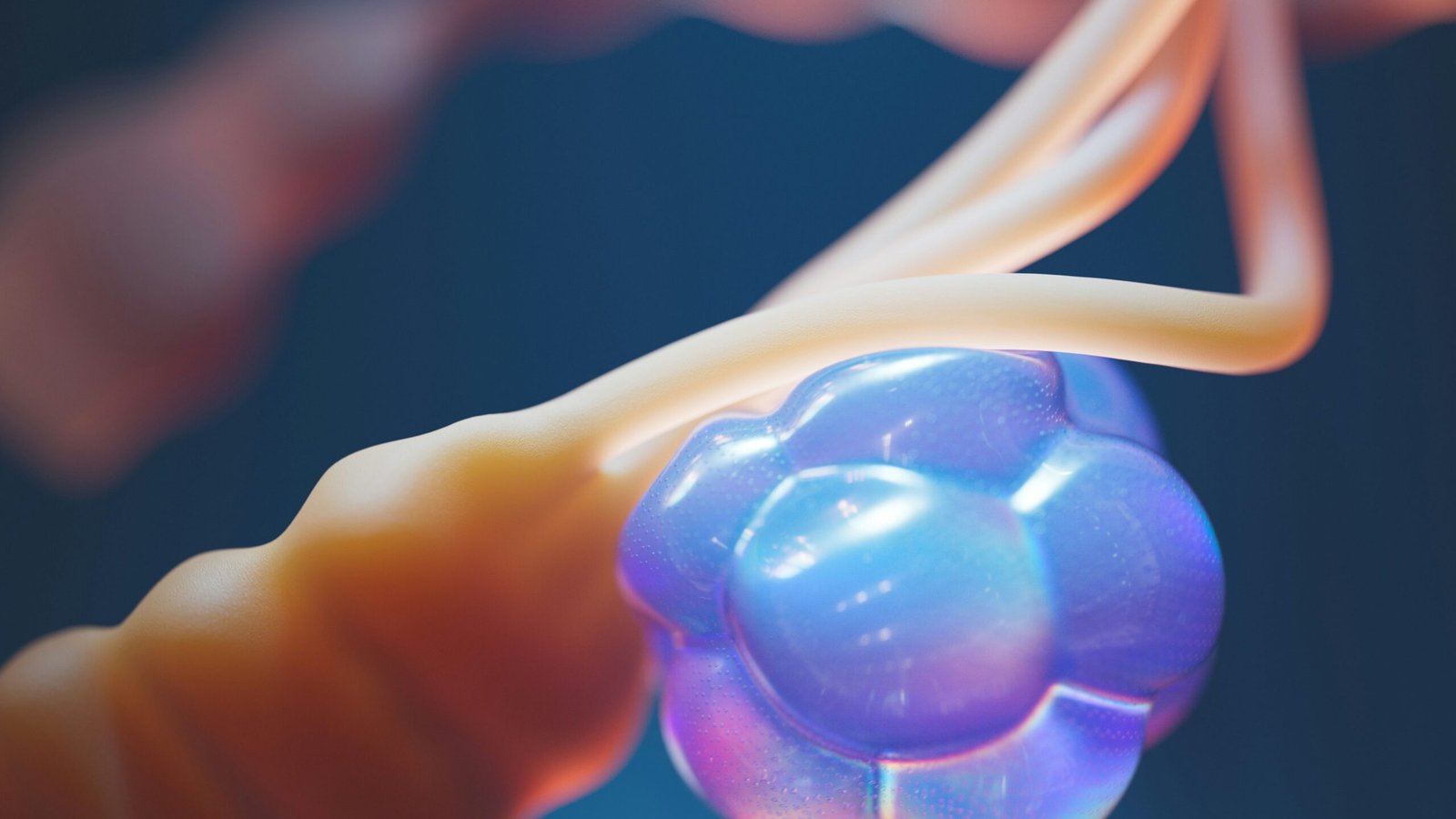
Introduction to Protein Folding and Its Importance
Protein folding is a fundamental biological process wherein a linear chain of amino acids folds into a three-dimensional structure. This intricate process is essential because the shape of a protein determines its function within the body. Proteins serve as enzymes, structural components, and signaling molecules, among other roles, and their activity is directly tied to their specific shapes. The complexity of protein structures arises from the vast number of possible folding configurations, making accurate prediction a formidable challenge.
Misfolded proteins can lead to severe diseases. For instance, in Alzheimer’s disease, aberrant folding results in the formation of amyloid plaques, which disrupt neural function. Similarly, in Parkinson’s disease, misfolded alpha-synuclein proteins aggregate into harmful structures called Lewy bodies. Cystic fibrosis is another example where a single misfolded protein, CFTR, fails to perform its function in the cell membrane, leading to severe respiratory and digestive issues. These examples underscore the critical importance of understanding protein folding mechanisms.
Historically, scientists have struggled to predict protein structures accurately due to the sheer complexity and variability of protein folding. Traditional methods like X-ray crystallography and nuclear magnetic resonance (NMR) spectroscopy, while effective, are time-consuming and labor-intensive. Computational approaches have shown promise, yet they often fell short in accuracy and efficiency. Overcoming these challenges is vital for biomedical research as it opens new avenues for drug discovery, understanding disease mechanisms, and developing novel therapies.
The advent of artificial intelligence (AI) in this realm marks a significant breakthrough. AI-powered models, such as those developed by DeepMind, have demonstrated remarkable accuracy in predicting protein structures. This progress heralds a new era in biomedical research, promising faster and more precise insights that could revolutionize our understanding of diseases and the development of treatments. The potential to accurately model protein folding could transform the landscape of medical science, offering hope for cures and improved therapies for various debilitating conditions.
The Role of AI in Predicting Protein Structures
Artificial intelligence has emerged as a formidable tool in the domain of protein folding, transforming the way scientists predict protein structures. The advent of machine learning algorithms has particularly revolutionized this field, with systems like AlphaFold and RoseTTAFold leading the charge. These AI-driven platforms have significantly enhanced both the accuracy and speed of protein structure predictions, offering unprecedented insights into the complex world of biomolecules.
The underlying technology propelling these advancements is deep learning. Deep learning algorithms excel at analyzing vast datasets, identifying intricate patterns, and making highly accurate predictions. In the context of protein folding, these algorithms process extensive biological data, including amino acid sequences and known protein structures, to predict the three-dimensional shapes of proteins. This capability is crucial because the shape of a protein determines its function, and understanding this relationship is fundamental to advancements in biomedical research.
AlphaFold, developed by DeepMind, is a prime example of how AI is revolutionizing protein folding. In 2020, AlphaFold achieved a significant breakthrough by accurately predicting the structures of proteins that had eluded scientists for decades. Using a deep learning model trained on a diverse array of protein structures, AlphaFold demonstrated a remarkable ability to predict protein shapes with a high degree of precision. This achievement was hailed as a milestone in computational biology, potentially accelerating the development of new drugs and therapies.
Similarly, RoseTTAFold, developed by the University of Washington, leverages deep learning to predict protein structures. RoseTTAFold has been instrumental in solving complex protein structures, aiding researchers in understanding diseases and developing treatments. By analyzing the relationships between protein sequences and structures, RoseTTAFold has provided valuable insights that were previously unattainable through traditional methods.
The impact of these AI systems extends beyond academia. They have the potential to revolutionize drug discovery, improve our understanding of genetic disorders, and pave the way for personalized medicine. By unlocking the secrets of protein folding, AI is not only accelerating biomedical research but also opening new frontiers in the quest to understand and treat human diseases.
Implications for Drug Discovery and Development
Advancements in AI-driven protein folding are significantly transforming the landscape of drug discovery and development. The accurate modeling of protein structures has become a cornerstone in understanding disease mechanisms, thereby facilitating the identification of potential drug targets. With the ability to predict protein shapes with unprecedented precision, researchers can now delve deeper into the molecular underpinnings of various diseases, paving the way for innovative therapeutic interventions.
By leveraging AI-generated protein models, pharmaceutical companies are increasingly able to design more effective and targeted therapies. These models provide crucial insights into the binding sites and interaction patterns of proteins, which are essential for the development of drugs that can precisely target disease-causing molecules. Consequently, this approach not only enhances the efficacy of new treatments but also minimizes adverse side effects, contributing to safer patient outcomes.
One of the most notable examples of AI’s impact in this field is the use of DeepMind’s AlphaFold. This groundbreaking AI system has revolutionized protein structure prediction, enabling researchers to solve complex protein configurations that were previously elusive. Pharmaceutical giants like Pfizer and GlaxoSmithKline have integrated AlphaFold’s capabilities into their drug discovery pipelines, accelerating the identification and validation of novel drug targets. Such advancements have the potential to drastically reduce the time and cost associated with bringing new drugs to market.
Ongoing projects continue to demonstrate the transformative power of AI in drug development. For instance, Insilico Medicine employs AI to predict the structure of proteins involved in aging and age-related diseases, leading to the identification of compounds that can modulate these targets. Similarly, Exscientia uses AI to optimize drug design and has successfully brought AI-designed molecules into clinical trials, showcasing the tangible benefits of this technology in accelerating therapeutic innovations.
The integration of AI in protein folding not only promises to revolutionize drug discovery processes but also holds the potential to address some of the most challenging biomedical questions of our time. As AI continues to evolve, its applications in this domain are poised to unlock new frontiers in the quest for effective and affordable healthcare solutions.
Future Prospects and Ethical Considerations
The future of AI in protein folding holds immense promise, with the potential to significantly advance biomedical research. As AI algorithms become increasingly sophisticated, they can provide more accurate and efficient solutions to complex biological problems. Future advancements in AI technology are expected to address current limitations, such as the prediction of protein structures with higher accuracy and the ability to handle larger and more diverse datasets. This could lead to breakthroughs in understanding diseases, drug discovery, and personalized medicine.
However, the integration of AI in protein folding raises several ethical considerations. One major concern is data privacy. As AI systems require vast amounts of data to function effectively, ensuring the privacy and security of sensitive biological data is paramount. Researchers and developers must implement robust data protection measures to prevent unauthorized access and misuse.
Another ethical issue is the potential for unequal access to AI technologies. Advanced AI tools and resources may be concentrated in well-funded institutions and developed countries, creating a disparity in research capabilities. To mitigate this, it is essential to promote equitable access to AI technologies across the global scientific community. Collaborative efforts and open-access initiatives can help bridge this gap, fostering a more inclusive approach to scientific advancements.
Maintaining scientific integrity is also crucial when incorporating AI in protein folding research. The reliability and reproducibility of AI-driven results must be rigorously validated. Transparent reporting of methodologies and findings will help ensure that AI-generated data is trustworthy and can be independently verified by the scientific community.
Ultimately, the responsible and equitable advancement of AI in protein folding will require collaborative efforts between AI researchers, biologists, and policymakers. By working together, these stakeholders can establish guidelines and frameworks that promote ethical practices, data privacy, and equal access. Such cooperation will be vital in harnessing the full potential of AI to revolutionize protein folding and drive forward biomedical research for the benefit of all.